Know The Basics Of Predictive Analysis
Know the basics of predictive analytics
Predictive analytics is about finding patterns in data for which you need a vast amount of the same. It involves using statistical algorithms and machine learning on a vast amount of data to draw patterns. It helps to predict future outcomes. With this objective data is drawn from existing as well as new data sets. So, historical data, as well as real-time data, are analyzed to come out with an assessment about the future.
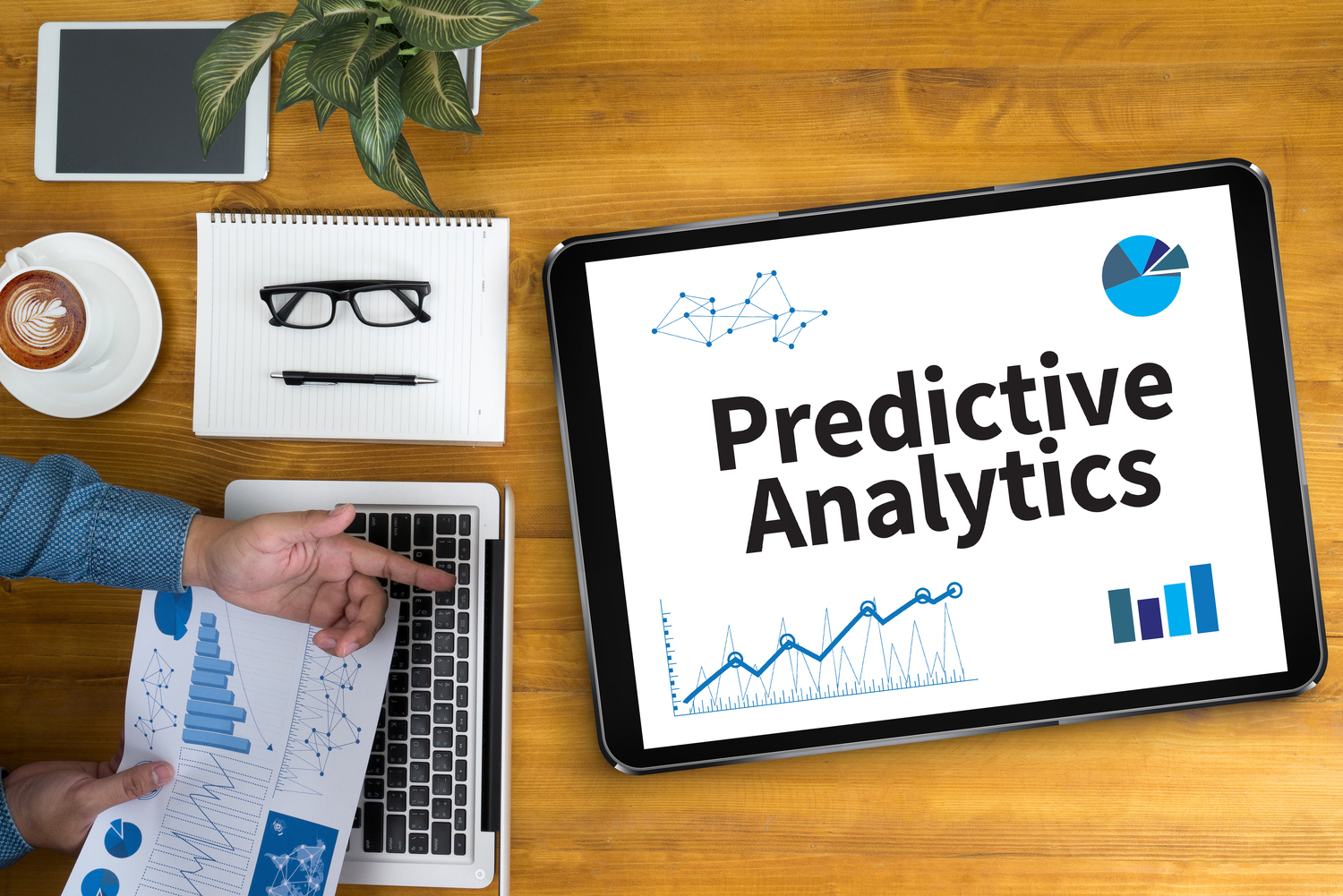
Companies have underscored the importance of predictive analytics. There are many companies which are using predictive analytics to predict different outcomes. The insurance industry, maritime organizations, app cab industry and many more are good examples predictive analytics can be used.
Why do companies resort to predictive analytics?
- Predictive analytics is important since it offers insight into various trends. It helps businesses to streamline their processes. It also helps companies to come up with changing trends and patterns in different niches.
- Predictive analytics helps in streamlining the marketing campaigns of companies by drawing patterns about customer purchases, customer response to various deals and promotions etc.
- It also helps companies to attract new customers and retain loyal customers. Companies also use predictive analytics in order to detect frauds. Some of the greatest examples predictive analytics use is in detecting frauds. It also helps improve business operations.
How do you start the exercise?
- The first step is to define your objective. Objectives vary from company to company and from industry to industry. You need to define the model objectively. It has to be relevant to the overall strategy and business goals of the company.
- There are several methods for developing objective models. Objectives may be modeled towards activation, response, retention, risk, lifetime value etc. Different models are applicable to different industries.
- You need to know which model is applicable for your kind of industry. However, that does not mean that there cannot be more than one model for one industry. In fact, there can be multiple objective models for each industry or company.
What is the next step in this process?
- The next step is gathering data. You need accurate, accessible and actionable data. The objective model depends on the accuracy, accessibility, relevance, and coherence of data.
- Organizations need to gather data about social interaction, product performance, new customer activities, app data etc. Every time a customer visits your website and does or does not make a purchase, it gathers more data.
- Some of this data may be with the website while some of it may be with third-party applications. If you can take all such data together, it is one of the greatest examples of predictive analytics.
What are the factors that determine the success of predictive analytics?
- The volume of data is very important. You need a vast amount of data to truly bring out a pattern in consumer or staff behavior.
- The speed at which this data is being fed into the system is also important. Real-time data like customer queries coming into the system need to be replied to in real-time. That creates a coherent set of actionable data. This will engage the customer effectively. This, in turn, can ensure that more data about customer behavior is obtained.
- The variety of data is also important. You need a variety of data to come to a conclusion. You need social media feeds, historical purchase data, data about store visits from customers, in-store video and more.
- The most important part of the data collection exercise is the veracity of data. If the data being fed into the system is not complete or false, it is not going to help you at all. If there are false accounts, social media trolls etc. your pursuit may be fruitless. Only when these four factors are taken into account can the system make an accurate predictive analytics.
What are the real-life examples predictive analytics?
- There are a variety of industries where predictive analytics is used. It is used in the travel industry to recommend hotels, flights, and ancillary services to new and regular customers. It is also used in the insurance industry to decide the rate of premium.
- It is used by various industries to customize the promotion of products and services. It is also used by social media to analyze sentiments, and for profiling users on various parameters.